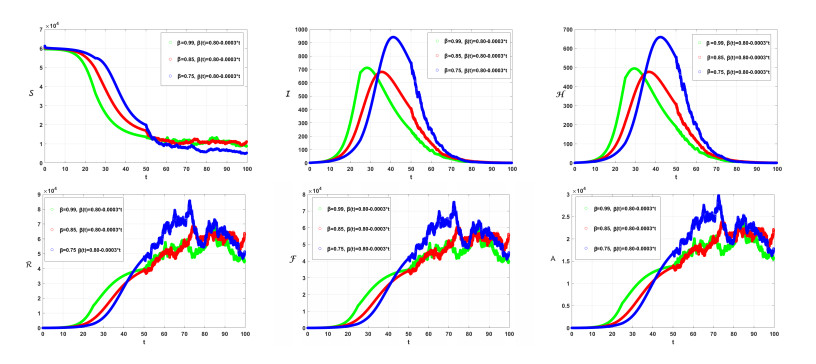
This paper extends a novel piecewise mathematical model of the COVID-19 epidemic using fractional and variable-order differential equations and fractional stochastic derivatives in three intervals of time. The deterministic models are augmented with hybrid fractional order and variable order operators, while the stochastic differential equations incorporate fractional Brownian motion. To probe the behavior of the proposed models, we introduce two numerical techniques: the nonstandard modified Euler Maruyama method for the fractional stochastic model, and the Caputo proportional constant-Grünwald-Letnikov nonstandard finite difference method for the fractional and variable-order deterministic models. Several numerical experiments corroborate the theoretical assertions and demonstrate the efficacy of the proposed approaches.
Citation: Fawaz K. Alalhareth, Seham M. Al-Mekhlafi, Ahmed Boudaoui, Noura Laksaci, Mohammed H. Alharbi. Numerical treatment for a novel crossover mathematical model of the COVID-19 epidemic[J]. AIMS Mathematics, 2024, 9(3): 5376-5393. doi: 10.3934/math.2024259
[1] | Mdi Begum Jeelani, Kamal Shah, Hussam Alrabaiah, Abeer S. Alnahdi . On a SEIR-type model of COVID-19 using piecewise and stochastic differential operators undertaking management strategies. AIMS Mathematics, 2023, 8(11): 27268-27290. doi: 10.3934/math.20231395 |
[2] | Muhammad Farman, Ali Akgül, Kottakkaran Sooppy Nisar, Dilshad Ahmad, Aqeel Ahmad, Sarfaraz Kamangar, C Ahamed Saleel . Epidemiological analysis of fractional order COVID-19 model with Mittag-Leffler kernel. AIMS Mathematics, 2022, 7(1): 756-783. doi: 10.3934/math.2022046 |
[3] | Rukhsar Ikram, Ghulam Hussain, Inayat Khan, Amir Khan, Gul Zaman, Aeshah A. Raezah . Stationary distribution of stochastic COVID-19 epidemic model with control strategies. AIMS Mathematics, 2024, 9(11): 30413-30442. doi: 10.3934/math.20241468 |
[4] | Murugesan Sivashankar, Sriramulu Sabarinathan, Vediyappan Govindan, Unai Fernandez-Gamiz, Samad Noeiaghdam . Stability analysis of COVID-19 outbreak using Caputo-Fabrizio fractional differential equation. AIMS Mathematics, 2023, 8(2): 2720-2735. doi: 10.3934/math.2023143 |
[5] | C. W. Chukwu, Fatmawati . Modelling fractional-order dynamics of COVID-19 with environmental transmission and vaccination: A case study of Indonesia. AIMS Mathematics, 2022, 7(3): 4416-4438. doi: 10.3934/math.2022246 |
[6] | Rahat Zarin, Amir Khan, Aurangzeb, Ali Akgül, Esra Karatas Akgül, Usa Wannasingha Humphries . Fractional modeling of COVID-19 pandemic model with real data from Pakistan under the ABC operator. AIMS Mathematics, 2022, 7(9): 15939-15964. doi: 10.3934/math.2022872 |
[7] | Maryam Amin, Muhammad Farman, Ali Akgül, Mohammad Partohaghighi, Fahd Jarad . Computational analysis of COVID-19 model outbreak with singular and nonlocal operator. AIMS Mathematics, 2022, 7(9): 16741-16759. doi: 10.3934/math.2022919 |
[8] | Aqeel Ahmad, Cicik Alfiniyah, Ali Akgül, Aeshah A. Raezah . Analysis of COVID-19 outbreak in Democratic Republic of the Congo using fractional operators. AIMS Mathematics, 2023, 8(11): 25654-25687. doi: 10.3934/math.20231309 |
[9] | Iqbal M. Batiha, Abeer A. Al-Nana, Ramzi B. Albadarneh, Adel Ouannas, Ahmad Al-Khasawneh, Shaher Momani . Fractional-order coronavirus models with vaccination strategies impacted on Saudi Arabia's infections. AIMS Mathematics, 2022, 7(7): 12842-12858. doi: 10.3934/math.2022711 |
[10] | Mohammad Sajid, Biplab Dhar, Ahmed S. Almohaimeed . Differential order analysis and sensitivity analysis of a CoVID-19 infection system with memory effect. AIMS Mathematics, 2022, 7(12): 20594-20614. doi: 10.3934/math.20221129 |
This paper extends a novel piecewise mathematical model of the COVID-19 epidemic using fractional and variable-order differential equations and fractional stochastic derivatives in three intervals of time. The deterministic models are augmented with hybrid fractional order and variable order operators, while the stochastic differential equations incorporate fractional Brownian motion. To probe the behavior of the proposed models, we introduce two numerical techniques: the nonstandard modified Euler Maruyama method for the fractional stochastic model, and the Caputo proportional constant-Grünwald-Letnikov nonstandard finite difference method for the fractional and variable-order deterministic models. Several numerical experiments corroborate the theoretical assertions and demonstrate the efficacy of the proposed approaches.
Despite significant advances in treatment and prevention technologies, contagious diseases continue to impact countless individuals globally. To limit the spread of these diseases, it is imperative to maintain strict control over factors such as transmission routes, population size, rates of human contact, duration of infection, and other critical parameters. Comprehending the behavior and management of pandemic diseases is particularly crucial during the initial infection phases or in the absence of immunization. In this context, mathematical models play an indispensable role. In recent years, scientists from various disciplines have delved into and researched numerous biological models, (see [1,2,3] and references therein).
The recent global health crisis, termed the COVID-19 pandemic, stems from the emergence of a novel coronavirus strain, SARS-CoV-2. This virus rapidly evolved into a significant worldwide concern. It has affected millions, placing an unparalleled burden on healthcare infrastructures and economies across the globe [4]. In the unyielding fight against this highly contagious and ever-evolving virus, mathematical modeling has proven indispensable. Offering quantitative tools to understand, simulate, and predict the virus's spread, these models have been instrumental in guiding public health measures and shaping policy decisions. Although mathematical modeling has been valuable in past epidemics, its significance has been magnified during the COVID-19 outbreak, where swift and informed decision-making has been crucial[5,6].
Many systems pertinent to real-world scenarios exhibit crossover behavior. Modeling systems based on this behavior has historically posed significant challenges. The transition from Markovian to non-Markovian processes has unveiled a plethora of examples that highlight real-world complexities. A prime illustration of this is in epidemiology, where the propagation of contagious diseases and occasional chaos may be attributed to this transition. Numerous intricate real-world challenges, including those involving chaos, have been articulated using the frameworks of piecewise integral and differential operators [7]. The cited authors delineated three distinct scenarios to frame deterministic-stochastic chaotic models and undertook numerical explorations of these constructs. Additionally, piecewise differentiation has emerged as a potent tool in epidemiological modeling, especially when challenges are presented by crossover dynamics [8,9].
Piecewise operators represent a novel class of operators established by Atangana et al. [10]. Traditional Mittag-Leffler or exponential mappings in fractional calculus need to provide a precise way to determine crossover time. A unique approach to piecewise derivation addressing this challenge was proposed in [10]. Piecewise differential equations offer insights into the present and serve as tools for future planning. Researchers employ these models to simulate diverse scenarios, such as potential virus resurgence and implications of new policy decisions. Active exploration of crossover behaviors with these operators is underway, with studies like [11,12] utilizing them to investigate qualitative characteristics of differential equations (DEs) and various infectious disease models. Further applications of piecewise operators are showcased in [13,14,15,16].
Atangana et al. [9] extended the concept of epidemiology modeling to portray waves with varying patterns, introducing a novel approach to ensure the existence and uniqueness of system solutions. They present a piecewise numerical approach for deriving solutions, with an illustrative example compared against data from Turkey, Spain, and Czechia, concluding that this method offers a new perspective for understanding natural phenomena. Zeb et al. [17] introduces a piecewise mathematical model for COVID-19 that incorporates a quarantine category and vaccination strategies. This model is based on the SEIQR framework for epidemic modeling. The authors in [18] developed a mathematical model using the Atangana–Baleanu fractional derivative to assess the Omicron variant of COVID-19, exploring strategies for reducing transmission risk. They expanded this model into a piecewise fractional stochastic Atangana–Baleanu differential equation, applying it to South African COVID-19 case data, and presented detailed numerical solutions and graphical results. The authors in [19] formulated a new mathematical model to investigate COVID-19 infection dynamics using Caputo fractional differential equations and piecewise stochastic differential equations. They present numerical solutions and graphical results for critical parameters, demonstrating that isolating healthy individuals from various stages of infected individuals can significantly reduce infection rates.
In this article, we introduce a COVID-19 model that comprises classes such as susceptible, exposed, symptomatic infectious, superspreaders (infectious but asymptomatic), hospitalized, recovery, and fatality. The model incorporates hybrid fractional order and variable order operators, and extends the stochastic differential equations with fractional Brownian motion. We introduce two numerical methods: the nonstandard modified Euler Maruyama method (NEMM) for solving the fractional stochastic model, and the Caputo proportional constant-Grünwald-Letnikov nonstandard finite difference method (CPC-GLNFDM) for the fractional and variable order deterministic models. Numerical simulations are conducted to validate the theoretical results and demonstrate the effectiveness of the proposed method. These experiments further provide insights into various phenomena, including crossover and stochastic processes.
The paper is structured as follows: Section 2 provides some basic concepts and preliminaries, formulation of the proposed model using the piecewise calculus, the existence and uniqueness of the model, and a numerical method for approximating the model's solutions. Numerical simulations, which validate the results from earlier sections, can be found in Section 3. In the end, Section 4 offers concluding observations.
Here, we define key terms from fractional calculus that will be consistently used in this study.
Definition 1. [20] Assuming f(τ) is a continuous function, given Ω=[μ,ν], −∞<μ<ν<+∞, β∈C, ℜ(β)>0. Below, the definitions are provided for the Riemann-Liouville derivatives, encompassing both the left and right variants, of order β:
μDβτf(τ)=1Γ(n−β)(ddτ)n∫τμf(s)(τ−s)1−n+βds, τ>μ, | (2.1) |
τDβνf(τ)=1Γ(n−β)(−ddτ)n∫ντf(s)(s−τ)1−n+βds, τ<ν | (2.2) |
where n=[ℜ(β)]+1.
Definition 2. [20,21] Let Ω=[μ,ν], where −∞<μ<ν<+∞, and let β∈C with ℜ(β)>0. The left and right Riemann-Liouville integrals of order β for a continuous function f(τ) are defined as:
RLμIβτf(τ)=μD−βτf(τ)=1Γ(β)[∫τμf(s)(τ−s)β−1ds], τ>μ, | (2.3) |
RLτIβνf(τ)=τD−βνf(τ)=1Γ(β)[∫ντf(s)(τ−s)β−1ds] τ<ν | (2.4) |
where 0<β<1.
Definition 3. [20] Within the field of complex numbers C, the descriptions of Caputo derivatives on the left and right sides of order β for a function f(τ) are given by
(CDβμ+f)(τ)=(CμDβτf)(τ)=1Γ(n−β)∫τμfn(s)(τ−ξ)1−n+βds, τ>μ, | (2.5) |
(CDβν−f)(τ)=(CτDβνf)(τ)=(−1)nΓ(n−β)∫ντfn(s)(s−τ)1−n+βds, τ<ν | (2.6) |
where n=[ℜ(β)]+1, ℜ(β)∉N0.
Definition 4. [22] In general, the operator known as the Caputo Proportional Fractional Hybrid (CP) operator is characterized as
CP0Dβτy(τ)=(∫τ0(y(s)K1(s,β)+y′(s)K0(s,β))(τ−s)−βds)1Γ(1−β),=(K1(τ,β)y(τ)+K0(τ,β)y′(τ))(τ−βΓ(1−β)) | (2.7) |
where K0(β,τ)=βτ(1−β), K1(β,τ)=(1−β)τβ, and 0<β<1.
Definition 5. [22] Fractional hybrid operators with a Caputo proportional constant (CPC) are described as
CPC0Dβτy(τ)=(∫τ0(τ−s)−β(y(s)K1(β)+y′(s)K0(β))ds)1Γ(1−β)=K1(β)RL0I1−βτy(τ)+K0(β)C0Dβτy(τ) | (2.8) |
where K0(β)=βQ(1−β), K1(β)=(1−β)Qβ are kernels Q is a constant, and 0<β<1.
Definition 6. [22] The variable-order fractional Caputo proportional operator (CP) is given as follows:
CP0Dβ(τ)τy(τ)=∫t0(Γ(1−β(τ)))−1(τ−s)−β(τ)(y′(s)K0(s,β(τ))+y(s)K1(s,β(τ)))ds,=(Γ(1−β(τ))−1τβ(τ))(y′(τ)K0(t,β(τ))+y(τ)K1(t,β(τ))). |
Here, K1(β(τ),τ)=(−β(τ)+1)τβ(τ),K0(β(τ),τ)=τ(1−β(τ))β(τ), and 1>β(τ)>0. Alternatively, the constant proportional Caputo (CPC) variable-order fractional hybrid operator can be formulated as follows:
CPC0Dβ(τ)τy(τ)=(∫t0(τ−s)−β(τ)1Γ(1−β(τ))(K1(β(τ))y(s)+y′(s)K0(β(τ)))ds)=K1(β(τ))RL0I1−β(τ)τy(τ)+K0(β(τ))C0Dβ(τ)τy(τ), |
where K0(β(τ))=Q(−β(τ)+1)β(τ), K1(β(τ))=Qβ(τ)(−β(τ)+1), Q is a constant, and 1>β(τ)>0. Moreover, its inverse operator is:
CPC0Iβ(τ)ty(t)=(∫τ0exp[K1(β(τ))K0(β(τ))(τ−s)]RL0D1−β(τ)τy(s)ds)1K0(β(τ)). |
The concept of a piecewise differential equation is utilized to expand upon the COVID-19 pandemic model initially introduced in [23]. We address potential dimensional incompatibilities by introducing an auxiliary parameter, ϑ, to the fractional model. Consequently, the proposed model segments the population into eight classes: susceptible (S), exposed (E), symptomatic and infectious (I), superspreaders (P), infectious but asymptomatic (A), hospitalized (H), recovery (R), and fatality (F). The corresponding piecewise (fractional-variable order-stochastic) differential equations for the COVID-19 epidemic are presented as follows:
{1ϑ1−βCPC0DβτS(τ)=−aIS−bHS−cPS,1ϑ1−βCPC0DβτE(τ)=aIS+bHS+cPS−dE,1ϑ1−βCPC0DβτI(τ)=−dE−fI,1ϑ1−βCPC0DβτP(τ)=−gE−λP,1ϑ1−βCPC0DβτA(τ)=μE,1ϑ1−βCPC0DβτH(τ)=v(P+I)−H+uH, 0<τ≤T1,0<β<1,1ϑ1−βCPC0DβτR(τ)=f(P+I)+H,1ϑ1−βCPC0DβτF(τ)=fI+P+uH | (2.9) |
with initial conditions
I(0)=I0≥0, S(0)=S0≥0,E(0)=E0≥0,A(0)=A0≥0,P(0)=P0≥0,R(0)=R0≥0,F(0)=F0≥0,H(0)=H0≥0. | (2.10) |
{1ϑ1−β(τ)CPC0Dβ(τ)τS(τ)=−aIS−bHS−cPS,1ϑ1−β(τ)CPC0Dβ(τ)τE(τ)=aIS+bHS+cPS−dE,1ϑ1−β(τ)CPC0Dβ(τ)τI(τ)=−dE−fI,1ϑ1−β(τ)CPC0Dβ(τ)τP(τ)=−gE−λP,1ϑ1−β(τ)CPC0Dβ(τ)τA(τ)=μE,1ϑ1−β(τ)CPC0Dβ(τ)τH(τ)=v(P+I)−H+uH, T1<τ≤T2,,0<β(τ)<11ϑ1−β(τ)CPC0Dβ(τ)τR(τ)=f(P+I)+H,1ϑ1−β(τ)CPC0Dβ(τ)τF(τ)=fI+P+uH, | (2.11) |
I(T1)=I1≥0, S(T1)=S1≥0,E(T1)=E1≥0,A(T1)=A1≥0,P(T1)=P1≥0,R(T1)=R1≥0,F(T1)=F1≥0,H(T1)=H1≥0. | (2.12) |
{dS=(−aIS−bHS−cPS)dt+σ1S(τ)dBH∗1,dE=(aIS+bHS+cPS−dE)dt+σ2E(τ)dBH∗2,dI=(−dE−fI)dt+σ3I(τ)dBH∗3,dP=(−gE−λP)dt+σ4P(τ)dBH∗4,dA=(μE)dt+σ5A(τ)dBH∗5,dH=(v(P+I)−H+uH)dt+σ6H(τ)dBH∗6, T2<τ≤Tf,dR=(f(P+I)+H)dt+σ7R(τ)dBH∗7,dF(τ)=(fI+P+uH)dt+σ8F(τ)dBH∗8, | (2.13) |
I(T2)=I2≥0, S(T2)=S2≥0,E(T2)=E2≥0,A(T2)=A2≥0,P(T2)=P2≥0,R(T2)=R2≥0,F(T2)=F2≥0,H(T2)=H2≥0 | (2.14) |
where σi and Bi(t), i=1,2,3,...,8 are the density of randomness and environmental noise, respectively, and H∗ is the Hurst index.
In the following, we demonstrate the existence and uniqueness of the system given by (2.9) and (2.10). However, before proceeding, it is essential to verify the conditions of Perov's theorem [24]. To do this, we first present preliminary results related to the Perov fixed point theorem and generalized Banach spaces. We refer the reader to [24] for a more detailed discussion.
Definition 7. Consider E as a vector space with K as its field, which could be R or C. In such a scenario, one can define a generalized norm as a function acting on E
‖⋅‖G: E⟶[0,+∞)nϕ↦‖ϕ‖G=(‖ϕ‖1⋮‖ϕ‖n) |
characterized by the subsequent properties
(i) For all ϕ∈E; if ‖ϕ‖G=0Rn+, then ϕ=0E,
(ii) ‖a‖G=|a|‖ϕ‖G for all ϕ∈E and a∈K, and
(iii) ‖ϕ+ω‖G≼‖ϕ‖G+‖ω‖G for all ϕ,ω∈E.
The pair (E,‖⋅‖G) defines a generalized normed space. If the vector-valued metric space is complete, the space \((E, \|\cdot\|_{G}) \) is termed a generalized Banach space (GBS), characterized by \(\delta_{G}(\phi_{1}, \phi_{2}) = \| \phi_{1}-\phi_{2}\|_{G}\).
Definition 8. Given a matrix Δ∈Mn×n(R+), it is considered to converge to zero when
Δm⟶On, as m⟶∞ |
where On is denoted as the zero n×n matrix.
Definition 9. Consider a generalized metric space \((E, \delta_{G}) \) and an operator \(N \) mapping \(E \) to itself. The operator \(N \) is termed a \(\varDelta \)-contraction with matrix \(\varDelta \) from \(\mathcal{M}_{n\times n}(\mathbb{R}_{+}) \) that tends towards \(\mathcal{O}_{n} \), provided that, for any \(\varrho, v \in E \), the following holds:
δG(N(ϱ),N(v))≼ΔδG(ϱ,v). |
The following result is Perov's extension of the Banach contraction principle.
Theorem 2.1. [24] Let E be a full generalized metric space and N:E⟶E be an operator that is an M-contraction. Then, N has a single fixed point in E.
Now, given that the systems (2.9) and (2.10) can be rewritten in the following classical form
{CPCDβτX(τ)=ϑ1−βF(X(τ)),X(0)=X0,0<τ<T1<∞, | (2.15) |
where, the vector X(τ)=(S0,E0,I0,P0,A0,H0,R0,F0)τ and the operator F is defined as follows
F(X)=(F1(X)F2(X)F3(X)F4(X)F5(X)F6(X)F7(X)F8(X))=(−aIS−bHS−cPSaIS+bHS+cPS−dE−dE−fI−gE−λPμEv(P+I)−H+uHf(P+I)+HfI+P+uH). | (2.16) |
Consider E=∏8i=1C([0,τ],R) to be a generalized Banach space when we endow it with the following generalized norm:
‖.‖G:E⟶R8+X↦‖X‖G=(‖S‖∞‖E‖∞‖I‖∞‖P‖∞‖A‖∞‖H‖∞‖R‖∞‖F‖∞). |
To show that systems (2.9) and (2.10) have a unique solution, we transform them into a fixed point problem for the operator N, defined by N:∏8i=1C([0,τ],R)→:∏8i=1C([0,τ],R),
N(X(τ))=X(0)+ϑ1−βK0(β)∫τ0exp(−K1(β)K0(β(τ))(τ−s))RL0D1−βτF(X(s))ds. | (2.17) |
The next lemma is needed.
Lemma 2.1. Assume a vector Δ∈R8 satisfies the given conditions
Δ=(Δ1Δ2Δ3Δ4Δ5Δ6Δ7Δ8)≽ϑ1−βΞmax(β)Ψmax(β)Γ(β−1)|K0(β)|(aΔ3Δ1+bΔ6Δ1+cΔ4Δ1|aΔ3Δ1+bΔ6Δ1+cΔ4Δ1−dΔ2|dΔ2fΔ3gΔ2λΔ4μΔ2|v(Δ4+Δ3)−Δ6+uΔ6|f(Δ4+Δ3)+Δ6fΔ3+Δ4+uΔ6). | (2.18) |
Then, the operator N maps the generalized ball ˉB(X0,Δ)⊂E into itself.
Next, we make sure that the operator F is G-Lipschitz.
Lemma 2.2. The operator F defined in (2.16) is a G-Lipschitz operator; i.e., there exists a square matrix ℧∈M6(R+) such that
‖F(X)−F(ˉX)‖G≼℧‖X−ˉX‖G. |
The proofs of the above two lemmas are stated in the Appendix. The next theorem ensures the existence and the uniqueness of the proposed model.
Theorem 2.2. Assuming that the matrix
ϑ1−βΞmax(β)Ψmax(β)Γ(β−1)|K0(β)|℧, | (2.19) |
converges to O8, then there is a unique solution for the initial value problem given by (2.9) and (2.10), and the solution holds for all τ>0 in ˉB(X0,Δ).
Proof. Further, we have for any X,ˉX∈ˉB(X0,Δ), and by using Lemma 2.2,
‖N(X)−N(ˉX)‖G=‖ϑ1−βK0(β)∫τ0exp(−K1(β)K0(β)(τ−s))(RL0D1−βτF(X(s))−RL0D1−βτF(ˉX(s)))ds‖G≼ϑ1−β|K0(β)|∫τ0|exp(−K1(β)K0(β)(τ−s))|‖RL0D1−βτF(X(s))−RL0D1−βτF(ˉX(s))‖Gds≼ϑ1−βΞmax(β)Γ(β−1)|K0(β)|‖∫τ0(τ−s)β−2(F(X(s))−F(ˉX(s)))ds‖G≼ϑ1−βΞmax(β)Ψmax(β)Γ(β−1)|K0(β)|‖F(X)−F(ˉX)‖G≼ϑ1−βΞmax(β)Ψmax(β)Γ(β−1)|K0(β)|℧‖X−ˉX‖G. |
Since the matrix ϑ1−βΞmax(β)Ψmax(β)Γ(β−1)|K0(β)|℧ converges to zero, the operator N is a G-contraction, and hence by applying Perov's fixed point theorem (2.1), systems (2.9) and (2.10) have only one solution in ˉB(X0,Δ).
We propose an effective discretization for the examined cross-over system using the Caputo proportional constant-Grünwald-Letnikov nonstandard finite difference method (CPC-GLNFDM). The approach we take to addressing the subsequent linear cross-over model (including fractional and variable order, both deterministic and stochastic) is outlined as follows:
(CPC0Dβτy)(τ)=ρy(τ), 0<τ≤T1, 0<β≤1, ρ<0 y(0)=y0 | (2.20) |
(CPC0Dβ(τ)τy)(τ)=ρy(τ), T1<τ≤T2, 0<β(τ)≤1, ρ<0 y(T1)=y1 | (2.21) |
y(τ)=(ρy(τ)+σy(τ)dBH∗(τ), T2<τ≤Tf, y(T2)=y2, | (2.22) |
where B(τ) represents the standard Brownian motion, σ, denotes the real constants or the intensity of the stochastic environment, and H∗ is the Hurst index.
The relation given by (2.8) can be expressed as follows:
CPC0Dβτy(τ)=1Γ(1−β)∫τ0(τ−s)−β(K1(β)y(s)+K0(β)y′(s))ds,=K1(β)RL0I1−βτy(τ)+K0(β)C0Dβτy(τ),=K1(β)RL0Dβ−1τy(τ)+K0(β)C0Dβτy(τ), | (2.23) |
where K1(β) and K0(β) are kernels depending solely on β and K0(β)=βQ(1−β), K1(β)=(1−β)Qβ, Q is a constant, and ω0=1. Using the GLNFDM approximation, (2.23) can be discretized as follows:
CPC0Dβτy(τ)|τ=τn=K1(β)(Θ(Δτ))β−1(yn+1+n+1∑i=1ωiyn+1−i)+K0(β)(Θ(Δτ))β(yn+1−n+1∑i=1μiyn+1−i−qn+1y0) | (2.24) |
where
Θ(Δτ)=Δτ+O(Δτ2),...,0<Θ(Δτ)<1,Δτ⟶0. |
Subsequently, (2.20) can be discretized as follows:
K1(β)(Θ(Δτ))β−1(yn+1+n+1∑i=1ωiyn+1−i)+K0(β)(Θ(Δτ))β(yn+1−n+1∑i=1μiyn+1−i−qn+1y0)=ρy(tn) | (2.25) |
where ω0=1, ωi=(1−βi)ωi−1, τn=n(Θ(Δτ)), Δτ=TfNn, Nn is a natural number. μi=(−1)i−1( βi), μ1=β, qi=iβΓ(1−β), and i=1,2,...,n+1.
In addition, we will proceed with the assumption that [25]
0<μi+1<μi<...<μ1=β<1, |
0<qi+1<qi<...<q1=1Γ(−β+1). |
Hence, if K1(β)=0 and K0(β)=1 in (2.25), then one has the discretization of the Caputo operator (C- GLNFDM) using the finite difference method.
Additionally, (2.21) can be discretized as follows:
K1(β(ti))(Θ(Δτ))β(ti)−1(yn2+1+n2+1∑i=n+1ωiyn2+1−i)+K0β(ti)(Θ(Δτ))β(ti)(yn2+1−n2+1∑i=n+1μiyn2+1−i−qn2+1y0)=ρy(tn2) | (2.26) |
where ωi=(1−β(ti)i)ωi−1, μi=(−1)i−1( β(ti)i), μ1=β(ti), qi=iβ(ti)Γ(1−β(ti)), and we can discretize (2.22) using the nonstandard modified Euler-Maruyama method (NMEMM) [26] as follows:
y(tn3+1)=y(tn3)+ρy(tn3)Θ(Δτ)+σy(tn3)ΔBn3+0.5y(tn3)Θ(Δτ)2H∗, H∗>0.5, n3=n2,...,κ, T2<τ≤Tf. |
To obtain the numerical solutions for the proposed piecewise models (2.9)–(2.14), we employ the following parameters: a=6.8262110−5, b=1.064810−4, c=2.047810−4, d=0.25, f=3.5, g=2.510−4, λ=2.21, μ=0.10475, v=0.94, u=0.3. The initial conditions are set as follows: S(0)=59659, I(0)=1, E(0)=0, P(0)=5, A(0)=0, R(0)=0, H(0)=0, R(0)=0, F(0)=0, and T1=25,T2=50, and Tf=100, Θ(Δτ)=1−e−Δτ. The numerical results for (2.9)-(2.13) are graphically represented at various values of \(\beta \), \(\beta(\tau) \), \(\sigma_{i} \) (where \(i \) ranges from 1 to 8), and \(H^{\ast}(\tau) \). The simulations are conducted using the nonstandard Euler-Maruyama method (NEMM) (2.27) and the Caputo proportional constant-Grünwald-Letnikov nonstandard finite difference method CPC-GLNFDM (2.26). The dynamical behavior of the systems given by (2.9)–(2.13) is illustrated in Figures 1 through 5. It is observed that the crossover behavior is prominent around the values \(T_{1} = 25 \), \(T_{2} = 50 \), and \(T_{f} = 100 \). After these points, the dynamics exhibit multiplicity in their behavior. Figure 1 illustrates the simulation for (2.9)–(2.13) with β(τ)=0.80−0.003t and H∗=0.7, considering various values of β and σi. Figure 2 demonstrates how the solutions behave under various scenarios, with different values of β(τ) and for β=0.8, and H∗=0.7. Figure 3 illustrates the change in the behavior of the solutions (2.9)–(2.13) when employing different values of H∗, β(τ)=0.75−0.001(cos2(τ/10)), and β=0.80,σi=0.05. Figure 4 illustrates the behavior of the solution of (2.9)–(2.13) for different values of H∗ and σi β(τ)=0.75−0.001(cos2(τ/10)) and β=0.80. To illustrate how parameter values σi and H influence the results, we refer to Figure 5. From the simulation, we discerned several nuanced insights of the model, which proved invaluable insights from both biological and mathematical perspectives.
In conclusion, a new mathematical model of piecewise fractional and variable-order differential equations and the fractional stochastic derivative of the COVID-19 epidemic has been developed. Moreover, two numerical techniques are constructed to solve the proposed model. These methods are the nonstandard modified Euler Maruyama method for the fractional stochastic model and the Caputo proportional constant-Grünwald-Letnikov nonstandard finite difference method for the fractional and variable-order deterministic models. Utilizing this approach has enriched our understanding of the dynamics underlying this intricate health crisis. Furthermore, by integrating fractional Brownian motion into stochastic differential equations, we have gleaned valuable insights into the unpredictable nature of infectious disease propagation. The development of two specialized numerical methods, namely, Caputo's proportional constant-Grünwald-Letnikov nonstandard finite difference method and the nonstandard modified Euler Maruyama technique, enabled us to delve deeply into the behavior of our extended models. Through a series of numerical tests, we have showcased the efficiency of these methods and garnered robust empirical support for our theoretical findings. Perhaps most crucially, our research has paved new pathways for studying the COVID-19 pandemic. The extended models afford the flexibility to examine a broad spectrum of behaviors, from deterministic patterns to stochastic processes. This allows for more adaptive strategies and interventions as circumstances change. This study stands as a substantial contribution to epidemiology, offering indispensable tools and insights that can guide public health decisions and aid in addressing the ongoing global health crisis.
The authors declare they have not used Artificial Intelligence (AI) tools in the creation of this article.
The authors are thankful to the Deanship of Scientific Research at Najran University for funding this work under the Research Priorities and Najran Research funding program grant code (NU/NRP/SERC/12/3).
The authors declare no conflicts of interest.
Proof of Lemma 2.1. Let X∈ˉB(X0,Δ), then
‖N(X)−X0‖G=‖ϑ1−βK0(β)∫τ0exp(−K1(β)K0(β)(τ−s))RL0D1−βτF(X(s))ds‖G≼ϑ1−β|K0(β)|∫τ0|exp(−K1(β)K0(β)(τ−s))|‖RL0D1−βτF(X(s))‖Gds≼ϑ1−βΞmax(β)Γ(β−1)|K0(β)|‖∫τ0(τ−s)β−2F(X(s))ds‖G≼ϑ1−βΞmax(β)Ψmax(β)Γ(β−1)|K0(β)|CF |
where
CF=supX∈ˉB(X0,Δ)‖F(X)‖G≼ϑ1−βΞmax(β)Ψmax(β)Γ(β−1)|K0(β)|(aΔ3Δ1+bΔ6Δ1+cΔ4Δ1|aΔ3Δ1+bΔ6Δ1+cΔ4Δ1−dΔ2|dΔ2fΔ3gΔ2λΔ4μΔ2|v(Δ4+Δ3)−Δ6+uΔ6|f(Δ4+Δ3)+Δ6fΔ3+Δ4+uΔ6) |
and this complete the proof.
Proof of Lemma 2.2. Let X=(S,E,I,P,A,H,R,F), ˉX=(ˉS,ˉE,ˉI,ˉP,ˉA,ˉH,ˉR,ˉF)∈ˉB(X0,Δ) and we have
|F1(X)−F1(ˉX)|=|−aIS−bHS−cPS+aˉIˉS+bˉHˉS+cˉPˉS|≤a|(ˉIˉS−IS)|+b|(ˉHˉS−HS)|+c|(ˉPˉS−PS)|. |
Also, we have for all ℘1,℘2,υ1,υ2∈R
|℘1υ1−℘2υ2|=12[(℘1−℘2)(υ1+υ2)+(℘1+℘2)(υ1−υ2)] |
and combining that with Lemma 2.1 we find
‖F1(X)−F1(ˉX)‖∞≤a2‖(ˉS−S)(ˉI+I)+(ˉI−I)(ˉS+S)‖∞+b2‖(ˉS−S)(ˉH+H)+(ˉH−H)(ˉS+S)‖∞+c2‖(ˉS−S)(ˉP+P)+(ˉP−P)(ˉS+S)‖∞≤a(Δ1‖ˉI−I‖∞+Δ3‖ˉS−S‖∞)+b(Δ1‖ˉH−H‖∞+Δ6‖ˉS−S‖∞)+c(Δ1‖ˉP−P‖∞+Δ4‖ˉS−S‖∞)≤Δ1(a‖ˉI−I‖∞+b‖ˉH−H‖∞+c‖ˉP−P‖∞)+(aΔ3+bΔ6+cΔ4)‖ˉS−S‖∞. | (4.1) |
Also, we have
‖F2(X)−F2(ˉX)‖∞=‖aIS+bHS+cPS−dE−aˉIˉS−bˉHˉS−cˉPˉS+dˉE‖∞≤Δ1(a‖ˉI−I‖∞+b‖ˉH−H‖∞+c‖ˉP−P‖∞)+d‖E−ˉE‖∞+(aΔ3+bΔ6+cΔ4)‖ˉS−S‖∞. |
The linearity of the operators F3,F4,F5, F6,F7, F8
‖F3(X)−F3(ˉX)‖∞≤d‖E−ˉE‖∞+f‖I−ˉI‖∞ |
‖F4(X)−F4(ˉX)|≤g‖E−ˉE‖∞+λ‖P−ˉP‖∞ |
‖F5(X)−F5(ˉX)|≤μ‖E−ˉE‖∞, |
‖F6(X)−F6(ˉX)‖∞≤v‖P−ˉP‖∞+v‖I−ˉI‖∞+|1−u|‖H−ˉH‖∞, |
‖F7(X)−F7(ˉX)‖∞≤f‖P−ˉP‖∞+f‖I−ˉI‖∞+‖H−ˉH‖∞, |
‖F8(X)−F8(ˉX)‖∞≤‖P−ˉP‖∞+f‖I−ˉI‖∞+u‖H−ˉH‖∞. |
By rewriting the above equations in matrix form we find
(‖F1(X)−F1(ˉX)‖∞‖F2(X)−F2(ˉX)‖∞‖F3(X)−F3(ˉX)‖∞‖F4(X)−F4(ˉX)‖∞‖F5(X)−F5(ˉX)‖∞‖F6(X)−F6(ˉX)‖∞‖F7(X)−F7(ˉX)‖∞‖F8(X)−F8(ˉX)‖∞)≼℧(‖S−ˉS‖∞‖E−ˉE‖∞‖I−ˉI‖∞‖P−ˉP‖∞‖A−ˉA‖∞‖H−ˉH‖∞‖R−ˉR‖∞‖F−ˉF‖∞) |
where
℧=((aΔ3+bΔ6+cΔ4)0aΔ1cΔ10bΔ100(aΔ3+bΔ6+cΔ4)daΔ1cΔ10bΔ1000df000000g0λ00000μ00000000vv0|u−1|0000ff010000f10u00). |
[1] |
F. K. Alalhareth, A. Boudaoui, Y. El hadj Moussa, N. Laksaci, M. H. Alharbi, Dynamic of some relapse in a giving up smoking model described by fractional derivative, Fractal Fract., 7 (2023), 573. https://doi.org/10.3390/fractalfract7070543 doi: 10.3390/fractalfract7070543
![]() |
[2] |
M. A. Khan, A. Atangana, Mathematical modeling and analysis of COVID-19: A study of new variant omicron, Phys. A: Statist. Mech. Appl., 599 (2022), 127452. https://doi.org/10.1016/j.physa.2022.127452 doi: 10.1016/j.physa.2022.127452
![]() |
[3] |
Y. Chang, M. Funk, S. Roy, E. Stephenson, S. Choi, H. V. Kojouharov, et al., Developing a mathematical model of intracellular calcium dynamics for evaluating combined anticancer effects of afatinib and rp4010 in esophageal cancer, Int. J. Mol. Sci., 23 (2022), 1763. https://doi.org/10.3390/ijms23031763 doi: 10.3390/ijms23031763
![]() |
[4] |
C. A. Pollard, M. P. Morran, A. L. Nestor-Kalinoski, The COVID-19 pandemic: A global health crisis, Physiol. Genom., 52 (2020), 549–557. https://doi.org/10.1152/physiolgenomics.00089.2020 doi: 10.1152/physiolgenomics.00089.2020
![]() |
[5] | H. H. Ayoub, H. Chemaitelly, S. Seedat, M. Makhoul, Z. Al Kanaani, A. Al Khal, et al., Mathematical modeling of the sars-cov-2 epidemic in qatar and its impact on the national response to COVID-19, J. Global Health, 11 (2021), 05005. https://doi.org/10.71892Fjogh.11.05005 |
[6] |
R. N. Thompson, Epidemiological models are important tools for guiding COVID-19 interventions, BMC Med., 18 (2020), 152. https://doi.org/10.1186/s12916-020-01628-4 doi: 10.1186/s12916-020-01628-4
![]() |
[7] |
N. H. Sweilam, S. M. AL-Mekhlafi, S. M. Hassan, N. R. Alsenaideh, A. E. Radwan, New coronavirus (2019-ncov) mathematical model using piecewise hybrid fractional order derivatives; numerical treatments, Mathematics, 10 (2022), 4579. https://doi.org/10.3390/math10234579 doi: 10.3390/math10234579
![]() |
[8] | A. Atangana, S. I. Araz, Deterministic-stochastic modeling: A new direction in modeling real world problems with crossover effect, HAL, 2021, 03201318. |
[9] |
A. Atangana, I. Koca, Modeling the spread of tuberculosis with piecewise differential operators, Comput. Model. Eng. Sci., 2021. http://dx.doi.org/10.32604/cmes.2022.019221 doi: 10.32604/cmes.2022.019221
![]() |
[10] |
A. Atangana, S. ˙I. Araz, New concept in calculus: Piecewise differential and integral operators, Chaos Solitons Fract., 145 (2021), 110638. https://doi.org/10.1016/j.chaos.2020.110638 doi: 10.1016/j.chaos.2020.110638
![]() |
[11] |
C. Xu, W. Alhejaili, S. Saifullah, A. Khan, J. Khan, M. El-Shorbagy, Analysis of huanglongbing disease model with a novel fractional piecewise approach, Chaos Solitons Fract., 161 (2022), 112316. https://doi.org/10.1016/j.chaos.2022.112316 doi: 10.1016/j.chaos.2022.112316
![]() |
[12] |
K. J. Ansari, Asma, F. Ilyas, K. Shah, A. Khan, T. Abdeljawad, On new updated concept for delay differential equations with piecewise caputo fractional-order derivative, Wave. Random Complex Media, 2023. https://doi.org/10.1080/17455030.2023.2187241 doi: 10.1080/17455030.2023.2187241
![]() |
[13] |
S. Saifullah, S. Ahmad, F. Jarad, Study on the dynamics of a piecewise tumor–immune interaction model, Fractals, 30 (2022), 2240233. https://doi.org/10.1142/S0218348X22402332 doi: 10.1142/S0218348X22402332
![]() |
[14] |
S. Naowarat, S. Ahmad, S. Saifullah, M. D. l. Sen, A. Akgül, Crossover dynamics of rotavirus disease under fractional piecewise derivative with vaccination effects: Simulations with real data from thailand, west africa, and the us, Symmetry, 14 (2022), 2641. https://doi.org/10.3390/sym14122641 doi: 10.3390/sym14122641
![]() |
[15] |
S. Ahmad, M. F. Yassen, M. M. Alam, S. Alkhati, F. Jarad, M. B. Riaz, A numerical study of dengue internal transmission model with fractional piecewise derivative, Results Phys., 39 (2022), 105798. https://doi.org/10.1016/j.rinp.2022.105798 doi: 10.1016/j.rinp.2022.105798
![]() |
[16] |
S. A. Abdelmohsen, M. F. Yassen, S. Ahmad, A. M. Abdelbacki, J. Khan, Theoretical and numerical study of the rumours spreading model in the framework of piecewise derivative, Eur. Phys. J. Plus, 137 (2022), 738. https://doi.org/10.1140/epjp/s13360-022-02921-2 doi: 10.1140/epjp/s13360-022-02921-2
![]() |
[17] |
A. Zeb, A. Atangana, Z. A. Khan, S. Djillali, A robust study of a piecewise fractional order COVID-19 mathematical model, Alex. Eng. J., 61 (2022), 5649–5665. https://doi.org/10.1016/j.aej.2021.11.039 doi: 10.1016/j.aej.2021.11.039
![]() |
[18] |
X. P. Li, M. H. DarAssi, M. A. Khan, C. Chukwu, M. Y. Alshahrani, M. Al Shahrani, et al., Assessing the potential impact of COVID-19 Omicron variant: Insight through a fractional piecewise model, Results Phys., 38 (2022), 105652. https://doi.org/10.1016/j.rinp.2022.105652 doi: 10.1016/j.rinp.2022.105652
![]() |
[19] |
X. P. Li, H. F. Alrihieli, E. A. Algehyne, M. A. Khan, M. Y. Alshahrani, Y. Alraey, et al., Application of piecewise fractional differential equation to COVID-19 infection dynamics, Results Phys., 39 (2022), 105685. https://doi.org/10.1016/j.rinp.2022.105685 doi: 10.1016/j.rinp.2022.105685
![]() |
[20] | I. Podlubny, Fractional Differential Equations: An Introduction to Fractional Derivatives, Fractional Differential Equations, to Methods of Their Solution and Some of Their Applications, Amsterdam: Elsevier, 1998. |
[21] |
A. Atangana, S. ˙I. Araz, Fractional derivatives andspecial functions, SIAM, 18 (1976), 240–268. https://doi.org/10.1137/1018042 doi: 10.1137/1018042
![]() |
[22] |
D. Baleanu, A. Fernandez, A. Akgül, On a fractional operator combining proportional and classical differintegrals, Mathematics, 8 (2020), 360. https://doi.org/10.3390/math8030360 doi: 10.3390/math8030360
![]() |
[23] |
A. Raza, D. Baleanu, T. N. Cheema, E. Fadhal, R. I. Ibrahim, N. Abdelli, Artificial intelligence computing analysis of fractional order COVID-19 epidemic model, AIP Adv., 13 (2023), 085017. https://doi.org/10.1063/5.0163868 doi: 10.1063/5.0163868
![]() |
[24] | A. Perov, On the cauchy problem for a system of ordinary differential equations, Pviblizhen, Met. Reshen. Differ. Uvavn, 2 (1964), 115–134. |
[25] | R. Scherer, S. L. Kalla, Y. Tang, J. Huang, The grünwald-letnikov method for fractional differential equations, Comput. Math. Appl., 62 (2011), 902–917. |
[26] | L. Y. Hu, Y, D. Nualart, Modified euler approximation scheme for stochastic differential equations driven by fractional brownian motions, preprint paper, 2013. https://doi.org/10.48550/arXiv.1306.1458 |
1. | N.H. Sweilam, S.M. Al-Mekhlafi, W.S. Abdel Kareem, G. Alqurishi, A new crossover dynamics mathematical model of monkeypox disease based on fractional differential equations and the Ψ-Caputo derivative: Numerical treatments, 2025, 111, 11100168, 181, 10.1016/j.aej.2024.10.019 | |
2. | Nasser H. Sweilam, Seham M. Al-Mekhlafi, Waleed S. Abdel Kareem, Ghader Alqurishi, Comparative Study of Crossover Mathematical Model of Breast Cancer Based on Ψ-Caputo Derivative and Mittag-Leffler Laws: Numerical Treatments, 2024, 16, 2073-8994, 1172, 10.3390/sym16091172 | |
3. | N.H. Sweilam, Waleed Abdel Kareem, S.M. ALMekhlafi, Muner M. Abou Hasan, Taha H. El-Ghareeb, T.M. Soliman, New Crossover Lumpy Skin Disease: Numerical Treatments, 2024, 26668181, 100986, 10.1016/j.padiff.2024.100986 | |
4. | Khaled Aldwoah, Hanen Louati, Nedal Eljaneid, Tariq Aljaaidi, Faez Alqarni, AbdelAziz Elsayed, Ahmad Al-Omari, Fractional and stochastic modeling of breast cancer progression with real data validation, 2025, 20, 1932-6203, e0313676, 10.1371/journal.pone.0313676 |